- unwind ai
- Posts
- Llama 4 Overpromises but Underdelivers
Llama 4 Overpromises but Underdelivers
PLUS: GPT-5 releasing this year, Vibe code with GitHub Copilot
Today’s top AI Highlights:
Llama 4 is here with overpromising release and underwhelming response
New full-featured MCP implementation with enterprise-grade auth
OpenAI gears up to release GPT-5 in a few months
Vibe code with GitHub Copilot’s new Agent mode
AI coding agent that can plan, write, and execute commands, with 2M context
& so much more!
Read time: 3 mins
AI Tutorials
Voice is the most natural and accessible way for users to interact with any application and we see it being used the most for customer support usecases. But building a voice agent that can access your knowledge base can be complex and time-consuming.
In this tutorial, we'll build a Customer Support Voice Agent using OpenAI's SDK that combines GPT-4o with their latest TTS (text-to-speech) model. Our application will crawl documentation websites, process the content into a searchable knowledge base, and provide both text and voice responses to user queries through a clean Streamlit interface.
We share hands-on tutorials like this every week, designed to help you stay ahead in the world of AI. If you're serious about leveling up your AI skills and staying ahead of the curve, subscribe now and be the first to access our latest tutorials.
Latest Developments
This was an unexpected weekend release — Meta just dropped their next generation of opensource multimodal models, Llama 4. The release includes two models, Scout and Maverick, both running with 17 billion active parameters but with different setups under the hood.
Llama 4 Scout comes with 16 experts (109B total parameters) and can fit on a single H100 GPU, while Maverick cranks it up with 128 experts (400B total parameters) and still delivers solid performance without needing a data center. Both handle text and images right out of the box, and Scout can process documents with a massive 10-million token context window. Meta's also teasing their heavyweight Behemoth model with 288B active parameters that they claim beats GPT-4.5 on STEM benchmarks, though it's still in training.
Scout and Maverick: The Models You Can Use Now - Scout is a 17B parameter model with 16 experts, that fits on a single NVIDIA H100 GPU. Maverick is a 17B parameter model with 128 experts, designed to outperform GPT-40 and Gemini 2.0 Flash in many categories. Both models are available for download on llama.com and Hugging Face.
Massive Context Window - Llama 4 Scout brings a massive 10M token context window, potentially changing what's possible with summarization, parsing, and reasoning across large datasets or codebases. According to Meta, a key architectural element is the introduction of interleaved attention layers along with inference time temperature scaling in order to support very long sequences.
Native Multimodal Integration - Both Scout and Maverick are built from the ground up for multimodality. Meta is touting advancements in early fusion techniques to seamlessly integrate text and vision tokens into a unified model backbone. Scout also comes with best-in-class image grounding for aligning user prompts with regions in the image for answering questions.
The Reality Check - While Meta’s benchmarks show Scout and Maverick outperforming Gemini 2.0 Pro, DeepSeek V3, and Claude 3.7 Sonnet, early community feedback is not that exciting. People are disappointed with Llama 4’s bad performance in areas like reasoning, coding, and long context comprehension. Meta might also catch itself in a controversy of mixing benchmark test sets in the post-training data.
You’ve heard the hype. It’s time for results.
After two years of siloed experiments, proofs of concept that fail to scale, and disappointing ROI, most enterprises are stuck. AI isn't transforming their organizations — it’s adding complexity, friction, and frustration.
But Writer customers are seeing positive impact across their companies. Our end-to-end approach is delivering adoption and ROI at scale. Now, we’re applying that same platform and technology to build agentic AI that actually works for every enterprise.
This isn’t just another hype train that overpromises and underdelivers. It’s the AI you’ve been waiting for — and it’s going to change the way enterprises operate. Be among the first to see end-to-end agentic AI in action. Join us for a live product release on April 10 at 2pm ET (11am PT).
Can't make it live? No worries — register anyway and we'll send you the recording!

MCP is gaining traction as the go-to standard for connecting AI tools with external data, with big players like OpenAI and Google showing interest. But while everyone's excited about its being the "REST for LLMs," the current spec lacks proper authentication mechanisms, forces each server to be its own identity provider, and primarily works with local connections rather than remote HTTP endpoints that enterprises need.
FeatureForm has released MCP Engine to tackle these issues head-on. MCP Engine is an open-source project that injects enterprise-grade security and scalability into MCP. It allows Claude and other LLMs talk to remote MCP servers while handling OAuth flows with providers like Okta and Google SSO behind the scenes. It is compatible with official MCP implementations while adding the security and scalability features that production environments demand.
Key Highlights:
Enterprise-ready authentication - MCPEngine solves MCP's authentication gap with standard OAuth integration for Okta, Google SSO, and other identity providers, eliminating the requirement for each MCP server to function as its own identity provider.
HTTP-first architecture - While the official MCP implementation prioritizes stdio-based local connections, MCPEngine employs a proxy system that maintains compatibility with LLM hosts like Claude Desktop while enabling proper HTTP-based server communications under the hood.
MCP Server Development - MCPEngine extends the official MCP SDK, simplifying the creation of secure MCP endpoints without resorting to workarounds. This means less time wrestling with the protocol and more time building cool stuff.
Backward Compatibility - MCPEngine ensures existing MCP integrations will continue to function smoothly. You won't need to rewrite your code to take advantage of the added security and scalability features.
Quick Bites
You can now vibe code with GitHub Copilot using their new Agent Mode, rolling out to all VS Code users. This upgrade lets Copilot actually take action across your codebase - working across multiple files, suggesting terminal commands, and even fixing runtime errors on its own. The agent mode achieves a pass rate of 56.0% on SWE-bench-verified with Claude 3.7 Sonnet. GitHub is also adding premium AI models from Anthropic, Google, and OpenAI to their lineup, with paid users getting a monthly quota of premium requests starting at 300 for Copilot Pro subscribers.
Sam Altman just revealed that OpenAI will drop o3 and o4-mini in the next couple of weeks, with GPT-5 finally coming in a few months. The flagship model is apparently taking longer because it's still training and being integrated with other features like Canvas and Deep Search. Altman claims they'll "make GPT-5 much better than we originally thought" - but we've all heard the hype before, so let's see if this is actually something special when it finally arrives.
Unsloth now supports fine-tuning Meta's new Llama 4 models with some impressive performance gains. Their optimizations make Llama 4 Scout training 1.5x faster and use 50% less VRAM than standard implementations, and context lengths 8x longer than environments using Flash Attention 2. They'll soon make Llama 4 Scout training fit in a single H100 80GB GPU once 4-bit support arrives. All Llama 4 versions (including dynamic 4-bit and 16-bit variants) are available on Hugging Face.
Google has moved Gemini 2.5 Pro into public preview with two-tier pricing, including significantly higher rate limits for paid users. The standard paid tier (Tier 1) is available immediately, while heavy users who've spent at least $250 and have been paying for 30+ days can access the beefier Tier 2 with substantially higher throughput limits. The API pricing ranges from $1.25 per million tokens for standard inputs to $15.00 per million tokens for larger outputs (over 200K tokens) with reasoning included.
The good news for free users is that nothing changes, as you can keep using the experimental version with the same model under the hood.
Tools of the Trade
GitHub MCP: GitHub has released its official opensource MCP Server that connects LLMs to GitHub APIs, letting them search repositories, manage issues, and create pull requests. It works with any MCP-compatible LLM client and runs locally with Docker.
Mirai iOS SDK: On-device AI inference engine that runs directly on iOS devices, supporting various model architectures including Llama, Gemma, Qwen, and VLMs. Comes with prompt analytics and dashboards, supports structured outputs.
Plandex: AI coding agent in your terminal that can plan and execute large coding tasks that span many steps and touch dozens of files. It can handle up to 2M tokens of context directly (~100k per file), and can index directories with 20M tokens or more using tree-sitter project maps. Completely opensource.
Exa Research Papers MCP: This MCP Server lets AI assistants use Exa's API to search over 100 million research papers, get their full content, and perform web searches with real-time results. Allows semantic search and full-text retrieval of academic content.
Awesome LLM Apps: Build awesome LLM apps with RAG, AI agents, and more to interact with data sources like GitHub, Gmail, PDFs, and YouTube videos, and automate complex work.
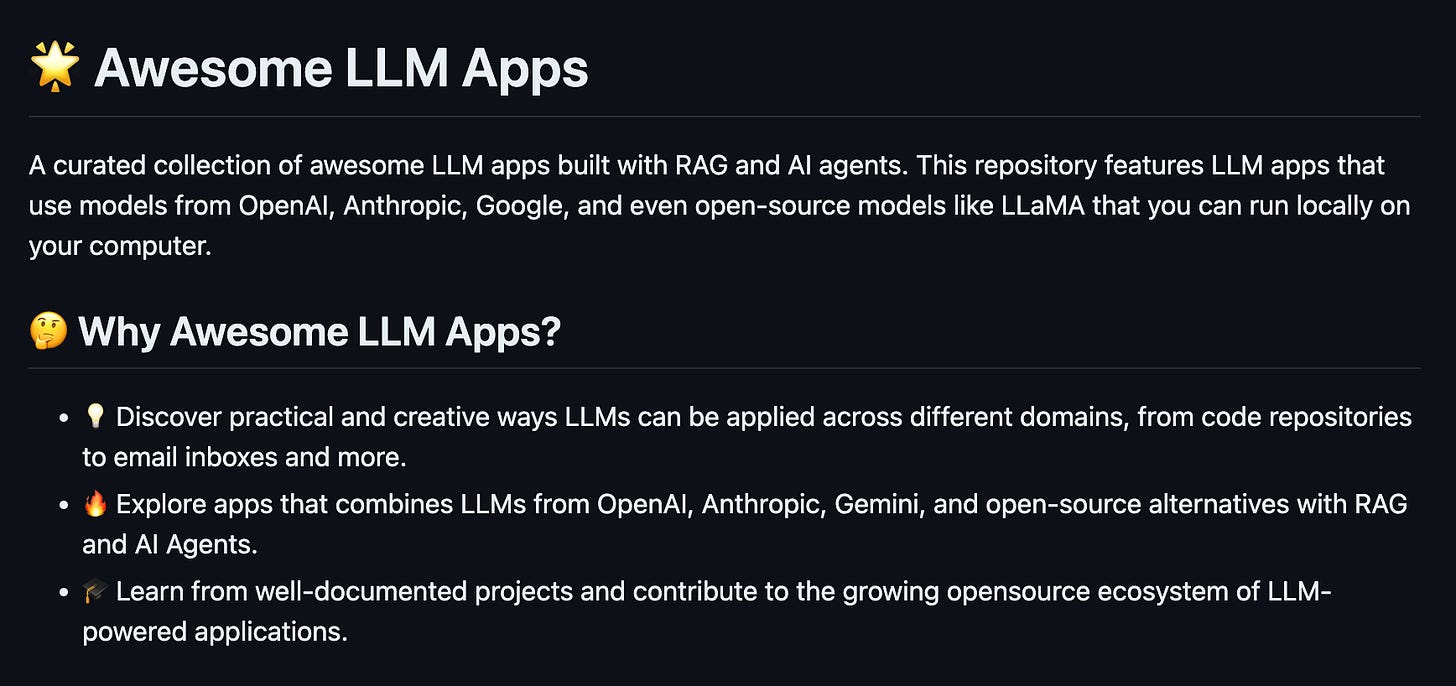
Hot Takes
My guess is that they launched Llama 4 on the weekend because they know that something big is coming this week. ~
Bojan Tunguzam i the only one that thinks you can prompt your way to agi easily but no one tries this because the current thing is working so why stop it ~
nearcyanHow you release models in 2025:
1. Train a model with some distinctive feature no one will ever be able to use or need.
2. Make sure it overfits to benchmarks so that you can call it competitive for its size.
3. Download the most recent Elo vote corpus and finetune to it to make sure you beat or equal the current leader.
4. Announce the model without creating a UI that allows anyone to test it. ~
Andriy Burkov
That’s all for today! See you tomorrow with more such AI-filled content.
Don’t forget to share this newsletter on your social channels and tag Unwind AI to support us!
PS: We curate this AI newsletter every day for FREE, your support is what keeps us going. If you find value in what you read, share it with at least one, two (or 20) of your friends 😉
Today’s top AI Highlights:
Build agentic RAG without similarity search, chunking, and vector DB
This all-in-one Superagent outperforms Manus AI and OpenAI Operator
Package and deploy your app on Windsurf to Netlify with a single click
Agent Swarms working in parallel is the new productivity multiplier
Stop Cursor, Claude or any LLM from generating broken, outdated code
& so much more!
Read time: 3 mins
AI Tutorials
Voice is the most natural and accessible way for users to interact with any application and we see it being used the most for customer support usecases. But building a voice agent that can access your knowledge base can be complex and time-consuming.
In this tutorial, we'll build a Customer Support Voice Agent using OpenAI's SDK that combines GPT-4o with their latest TTS (text-to-speech) model. Our application will crawl documentation websites, process the content into a searchable knowledge base, and provide both text and voice responses to user queries through a clean Streamlit interface.
We share hands-on tutorials like this every week, designed to help you stay ahead in the world of AI. If you're serious about leveling up your AI skills and staying ahead of the curve, subscribe now and be the first to access our latest tutorials.
Latest Developments

Your domain experts know that context matters more than keyword matching and vector-based similarity search isn’t enough. Vectify AI just open-sourced PageIndex, a new document indexing system built for reasoning-based RAG. It structures lengthy PDFs into semantic trees - think of it as a smart table of contents that helps LLMs find exactly what they need.
This approach was inspired by tree search algorithms similar to those in AlphaGo, making it particularly effective for domain-specific content where traditional embedding models struggle.
Key Highlights:
No Vector Chunks - PageIndex organizes content hierarchically based on document structure, eliminating arbitrary chunking and preserving natural section boundaries. This makes it especially effective for professional documents where context matters and similar terms might confuse vector-based systems.
Precise Page Referencing - Each node contains a summary along with exact physical page numbers, allowing your application to retrieve and cite specific information with pinpoint accuracy – critical for professional domains like finance, legal, or technical documentation.
No Vector Database Required - The system stores document trees in standard databases, significantly reducing infrastructure complexity while making it easier to integrate expert knowledge and user preferences into the retrieval process.
Performance Where It Counts - In benchmarks like FinanceBench, reasoning-based retrieval using PageIndex achieved 98.7% accuracy for financial document analysis, outperforming traditional vector-based approaches in domain-specific applications.

This is a new generation of AI agents that can autonomously think, plan, use a computer, and complete any task for you. What started with Anthropic Computer Use, OpenAI Operator, and Manus AI, we’re now seeing a wave of these agents, releasing every other, each showing improvements in end-to-end handling of complex, multi-step workflows.
Here’s another one that made our jaws drop after Manus AI. Genspark is an ultimate all-in-one super agent that can think, plan, act, and use tools to handle all your everyday tasks. Think about travel planning with your special preferences, conducting research, or even making a phone call for a reservation!
But Genspark is very different from how the other computer use agents work. Rather than using a computer in a sandboxed VM, it uses its in-house system to directly call relevant APIs whenever it needs to perform an action.
Key Highlights:
Mixture-of-Agents System - The Super Agent doesn't rely on just one model; it utilizes a network of 9 different-sized LLMs to best suit particular tasks to optimize for the task requirements and reduce potential error and hallucination.
In-House Toolsets - It has access to 80+ pre-built and tested toolsets to best suit particular tasks. As the agent thinks and plans through the task given, it autonomously calls and uses these tools as needed. This allows for better integration with tasks like travel and restaurant bookings.
In-House Datasets - It is embedded with pre-built datasets distilled from the web, guaranteeing data quality and freshness, which is a crucial thing to consider for devs working with real time data and task managements.
Fast, Accurate, & Steerable - The Super Agent provides near-instant results and control over outputs. Because it uses direct integrations, responses can be refined and deployed faster. As for the accuracy, it outperforms OpenAI Operator, Manus AI, and other SOTA agents on the GAIA benchmark.
You can try Genspark Super Agent for free right now.

Cognition AI just rolled out Devin 2.0, the latest iteration of its AI software engineer, introducing a completely redesigned agent-native IDE experience at a new starter price of just $20. The update enables you to run multiple autonomous Devin instances simultaneously while interacting with them through a familiar VSCode-like environment. Devin 2.0 also brings significant efficiency improvements, with each Agent Compute Unit (ACU) now delivering 83% more completed tasks than previous versions, making AI-assisted development more accessible to individual developers and small teams.
Key Highlights:
Multi-Agent Collaboration - Developers can now spin up parallel Devin instances to tackle multiple tasks concurrently, each with its own cloud-based IDE and isolated environment, allowing for easy context switching between different development tasks.
Interactive Planning System - Before execution, Devin proactively analyzes your codebase and presents relevant files, findings, and an initial plan within seconds - letting you refine the approach before implementation begins rather than starting with detailed requirements documents.
Codebase Understanding Tools - The new Devin Search feature enables developers to query their codebase directly with cited answers, while Deep Mode supports complex exploration questions that require extensive repository analysis.
Automatic Documentation - Devin Wiki automatically indexes repositories every few hours, generating architecture diagrams, documentation, and source links - addressing the persistent challenge of keeping technical documentation synchronized with rapidly evolving codebases.
Quick Bites
Lindy AI, the platform to build AI agents and automation, has released Agents Swarm that puts a swarm of AI agents to work simultaneously. A workflow is broken down into multiple sub-tasks. Lindy creates multiple copies of itself, with each sub-task assigned to an agent. These agents work simultaneously to complete a complex multi-step workflow in seconds.
Taking the same concept a notch up, Convergence AI has released Parallel Agents for Proxy, where multiple computer-use agents work simultaneously to do the task at hand. Once a task is given, a planning agent breaks it down to sub-tasks, Proxy spins up multiple agents within the browser, with each agent assigned a sub-task. You can see these agents navigating their own browsers, all together, completing the tasks insanely fast. It seems agents swarms is the next best thing!
Windsurf has introduced a new "Deploys" feature that allows you to package and share your applications to a public domain through Netlify integration, with just a single click. Wave 6 also brings enterprise access to Model Context Protocol and Turbo Mode, adds commit message generation, and improves conversation management with a new Table of Contents feature for easier navigation.
Anthropic is holding its first-ever developer conference, Code with Claude, on May 22 in San Francisco. Code with Claude is a hands-on, one-day event focused on exploring real-world implementations and best practices using the Anthropic API, CLI tools, and MCP. It is open to a select group of developers and founders. Apply here to attend.
PayPal has launched an MCP Server for merchants to do business tasks like creating invoices seamlessly using Claude, Cursor, Cline, and other MCP clients. Available both as a local installation and as a remote service that maintains sessions across devices, this integration brings the power of conversational AI to PayPal's business tools, while maintaining secure authentication with PayPal accounts..
Tools of the Trade
Context7: Provides up-to-date, version-specific documentation and code examples to LLMs, preventing them from generating outdated or incorrect code. It sources information directly from official documentation, filters it for relevance, and delivers it to AI assistants like Cursor or Claude. Completely free for personal use. Support for MCP servers and APIs coming soon.
Arrakis: A fully customizable and self-hosted sandbox for AI agent code execution and computer use. It features out-of-the-box support for backtracking, a simple REST API and Python SDK, automatic port forwarding, and secure MicroVM isolation. Perfect for safely running, testing, and backtracking multi-step agent workflows.
VibeCode: The OG vibe coder on X, Riley Brown has released this app that builds mobile apps from simple text descriptions. Just type in their idea and the app turns into a functioning app, which you can further edit through simple prompts.
Awesome LLM Apps: Build awesome LLM apps with RAG, AI agents, and more to interact with data sources like GitHub, Gmail, PDFs, and YouTube videos, and automate complex work.
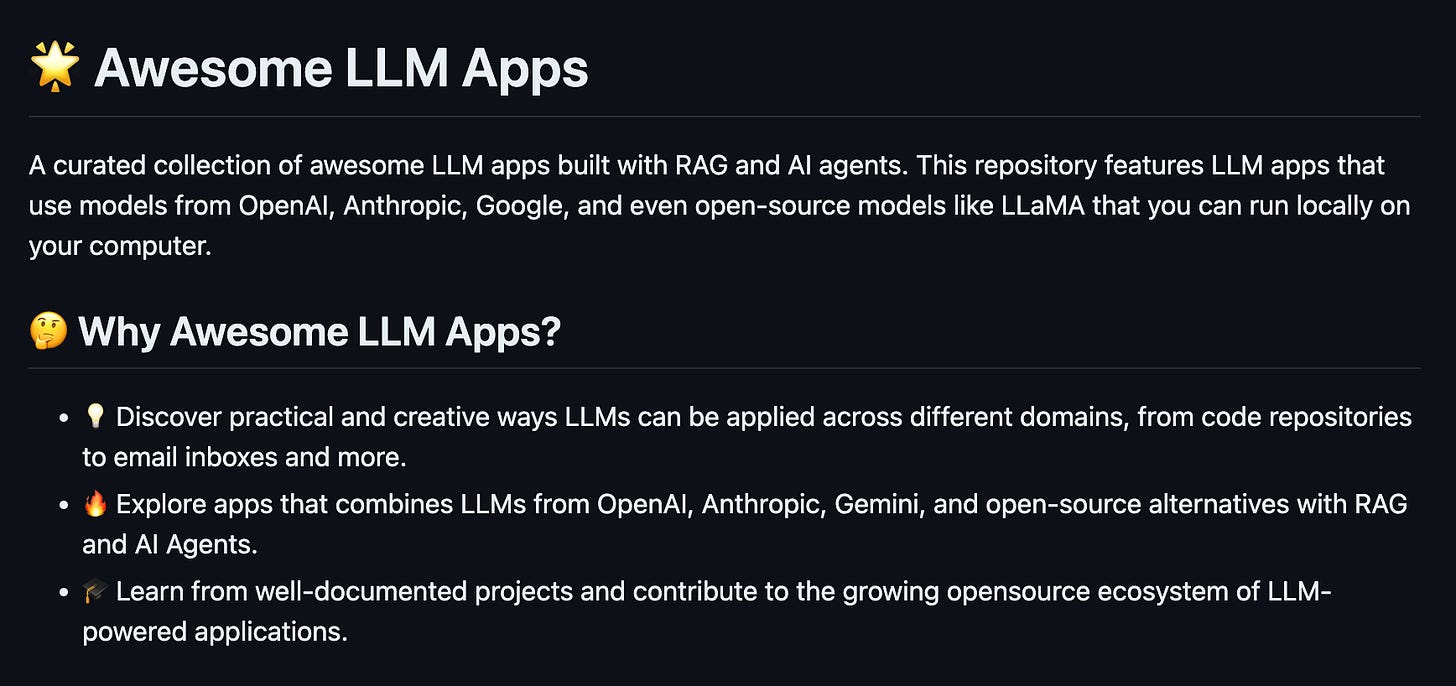
Hot Takes
The biggest mistake in vibe coding is prompting the agent to fix errors instead of rolling back
Hallmark sign of a junior vibe coder ~
Tom DörrOAI moat is until Deepseek drop the GOAT image gen model. Let them enjoy their few months of glory ( truly deserved for their hardwork but they are robbing people with pricing ) ~
Shi
That’s all for today! See you tomorrow with more such AI-filled content.
Don’t forget to share this newsletter on your social channels and tag Unwind AI to support us!
PS: We curate this AI newsletter every day for FREE, your support is what keeps us going. If you find value in what you read, share it with at least one, two (or 20) of your friends 😉
Reply